1 - Asus VivoBook 16 X1605ZA-MB310
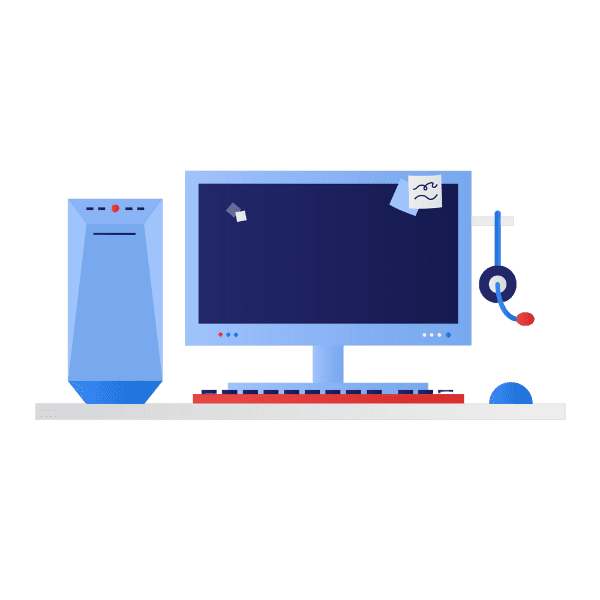
O Asus VivoBook 16 X1605ZA-MB310 se destaca no universo Linux por várias razões. Primeiro, sua compatibilidade nativa com o sistema operacional Linux, permitindo que os usuários desfrutem de uma experiência otimizada e livre de problemas com drivers ou compatibilidade de software.
Além disso, o hardware deste modelo é notavelmente potente, equipado com processadores de última geração e uma memória RAM robusta, garantindo uma operação suave mesmo em tarefas intensivas.
Sua tela de 16 polegadas oferece uma área de visualização ampla, ideal para profissionais de criação de conteúdo e programadores que precisam de mais espaço na tela.
A escolha do Asus VivoBook 16 X1605ZA-MB310 reflete um equilíbrio perfeito entre desempenho, durabilidade e preço, tornando-o uma opção excelente para usuários Linux em 2024.
2 - Lenovo V14 82ULS00200
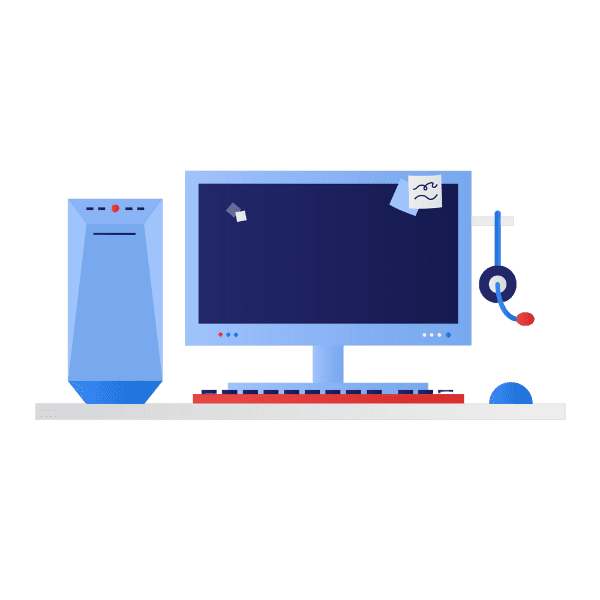
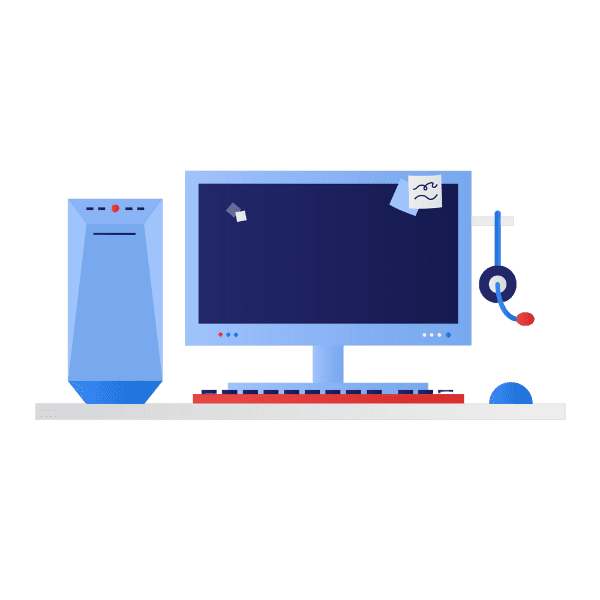
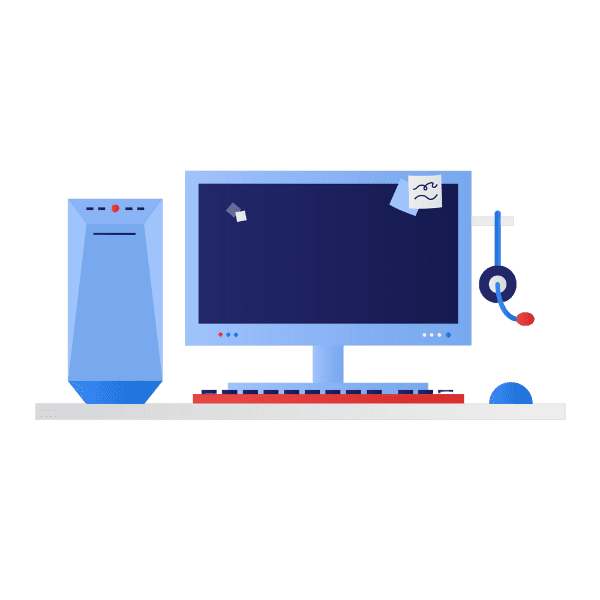
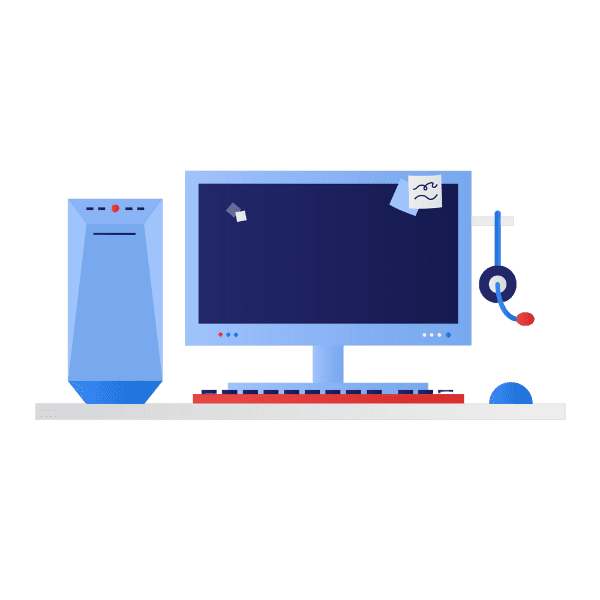
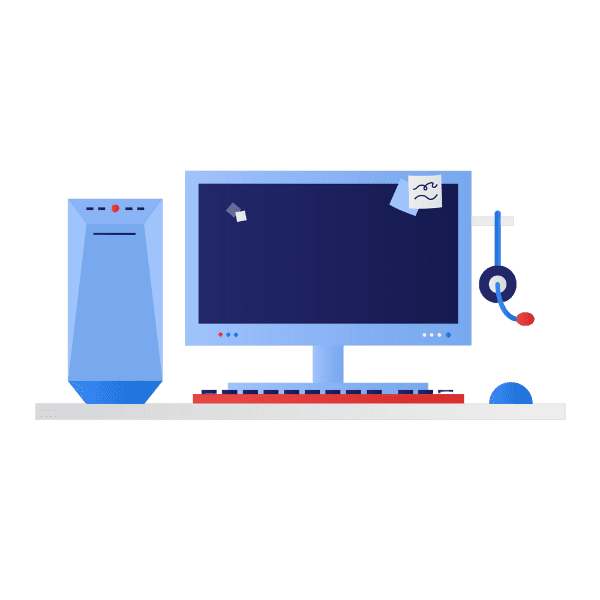
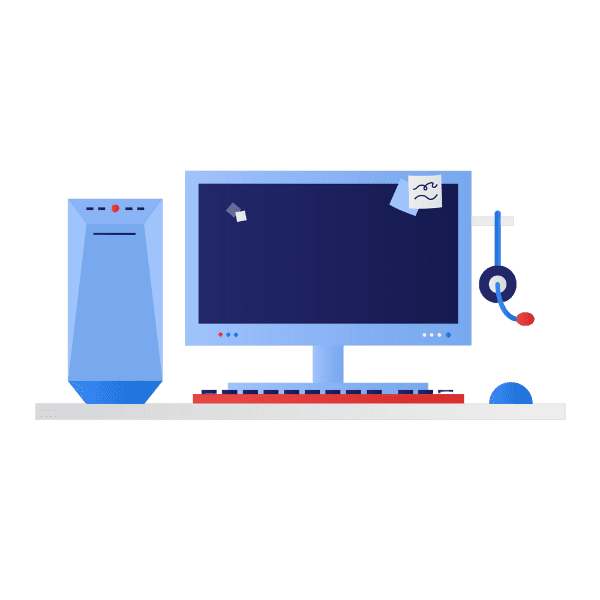
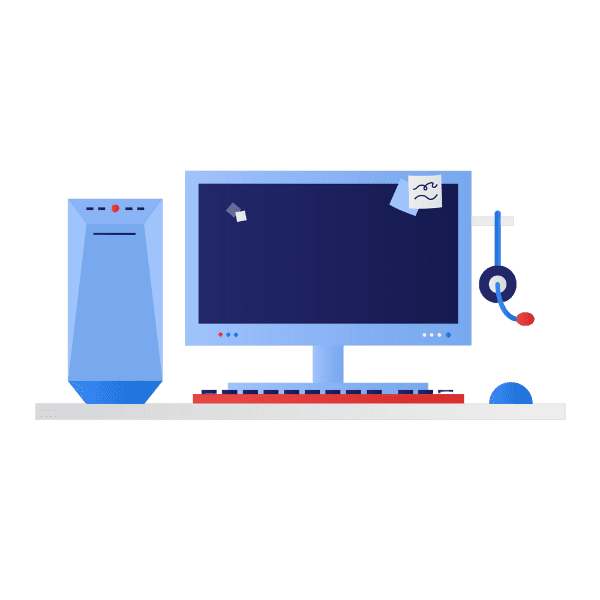
O Lenovo V14 82ULS00200 se destaca no mercado de notebooks Linux principalmente pela sua excelente portabilidade. Projetado pensando nos usuários que estão sempre em movimento, este modelo é incrivelmente leve e fino, facilitando o transporte sem sacrificar o desempenho. Ele vem equipado com um processador eficiente que garante uma operação suave para tarefas do dia a dia, além de oferecer uma bateria de longa duração que é ideal para aqueles longos dias fora de casa ou do escritório.
A tela de alta definição proporciona imagens claras e nítidas, tornando-o adequado tanto para trabalho quanto para entretenimento.
Além disso, sua compatibilidade com o Linux significa que os usuários podem aproveitar uma experiência de software segura e personalizável, com acesso a uma vasta biblioteca de aplicativos gratuitos.
Este notebook é uma escolha excelente para profissionais e estudantes que precisam de um dispositivo confiável e fácil de transportar, sem comprometer o acesso às poderosas ferramentas que o Linux tem a oferecer.
3 - Lenovo IdeaPad 3 82MFS00600
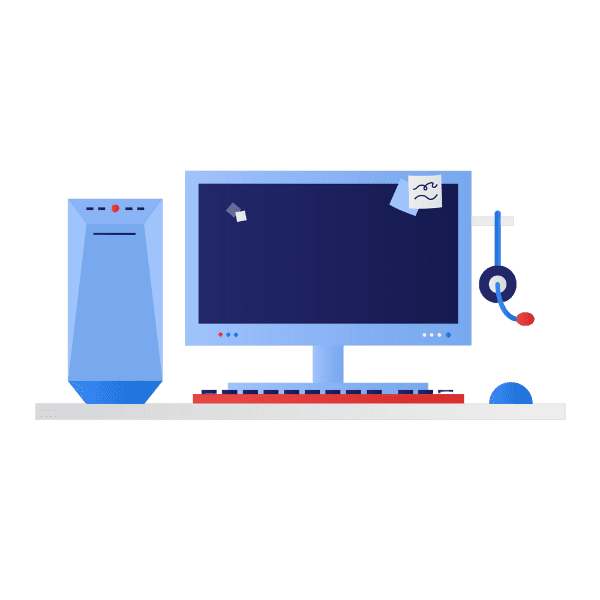
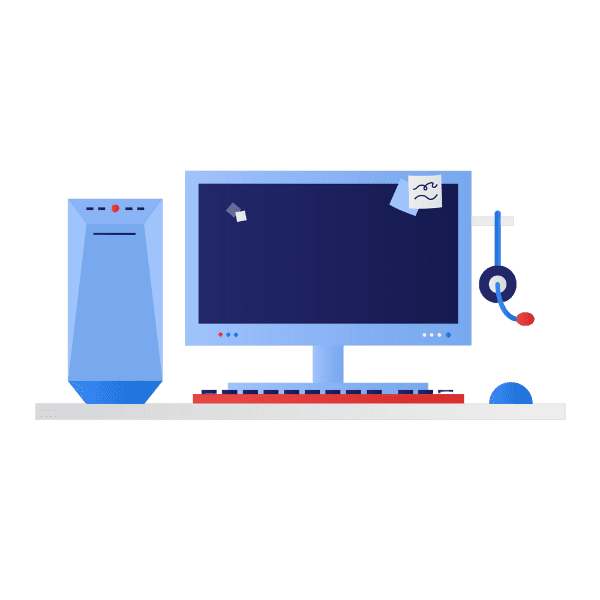
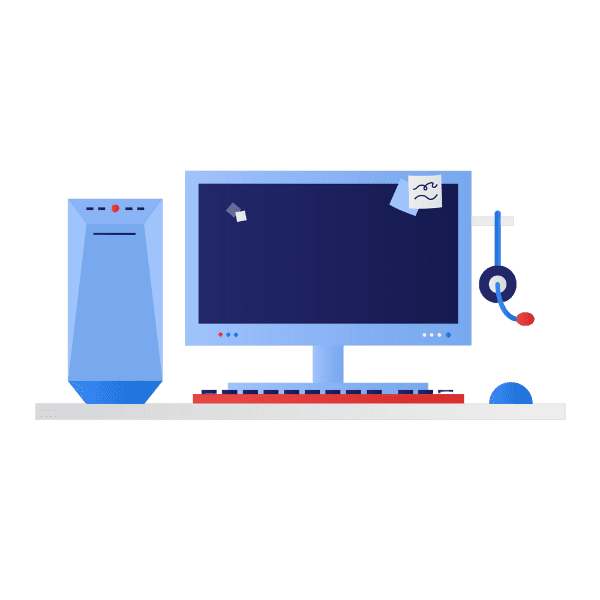
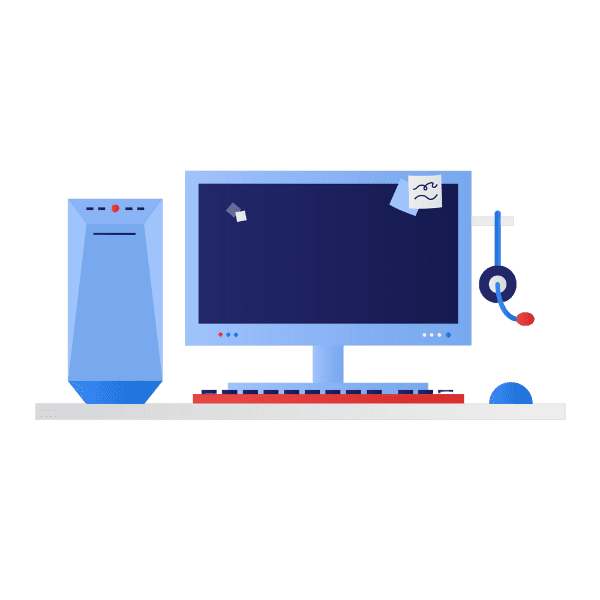
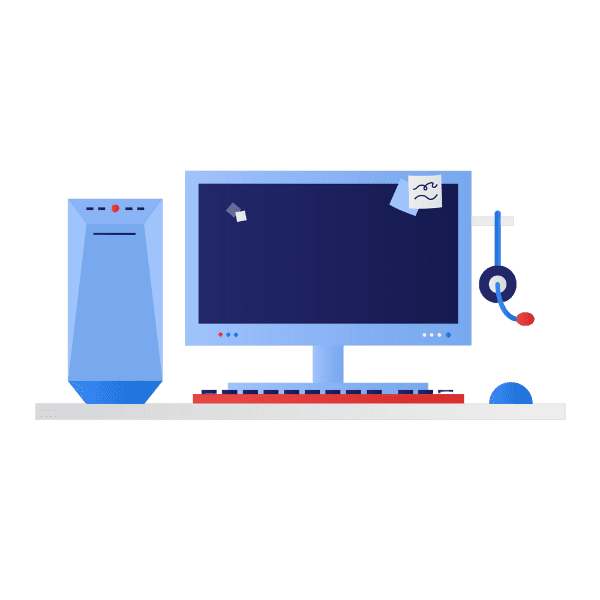
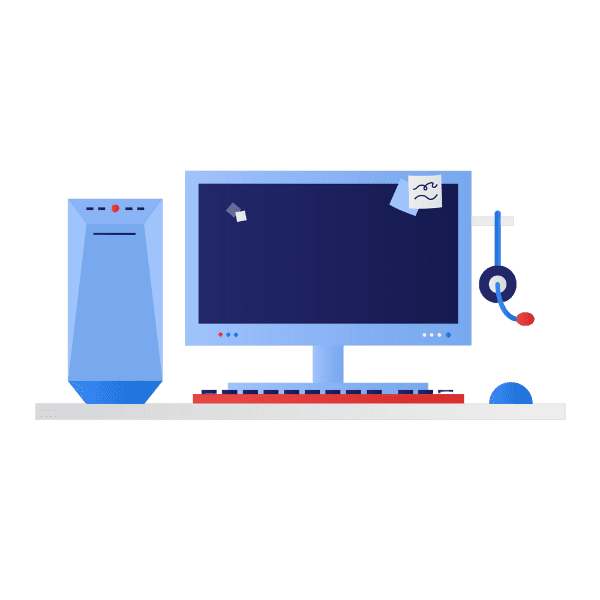
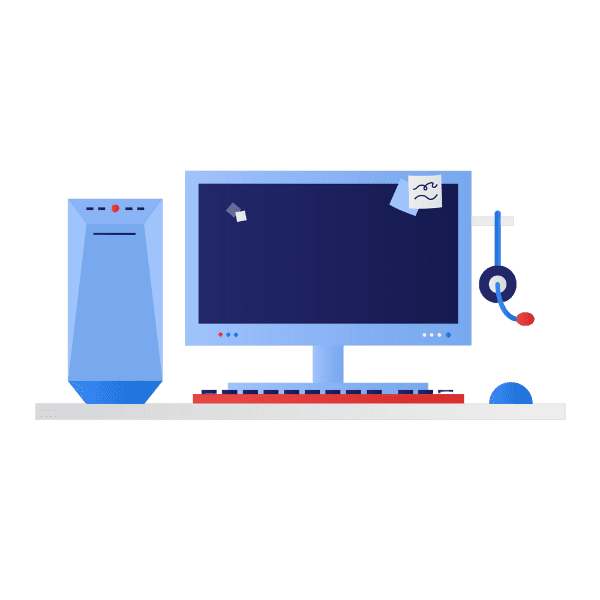
O Lenovo IdeaPad 3 82MFS00600 é uma escolha soberba para usuários que necessitam de uma máquina capaz de lidar com múltiplas tarefas simultaneamente sem desacelerar, graças à sua excelente memória RAM.
Equipado com uma quantidade generosa de RAM, este modelo é ideal para desenvolvedores, designers gráficos e jogadores que precisam executar aplicativos e softwares exigentes.
Além de sua capacidade de memória robusta, o IdeaPad 3 oferece um processador de alta performance que complementa perfeitamente a RAM, assegurando uma experiência de usuário suave e eficiente.
A tela de alta resolução fornece imagens vívidas e detalhadas, o que é uma vantagem adicional para profissionais criativos e entusiastas de mídia. Compatível com o Linux, este notebook não apenas promete uma operação rápida e responsiva, mas também oferece a segurança e flexibilidade que os usuários do sistema operacional aberto valorizam. Seja para trabalho intensivo de programação, design gráfico ou para lazer, o Lenovo IdeaPad 3 82MFS00600 se destaca como uma máquina versátil e potente.
4 - Acer Nitro 5 AN515-58-58W3
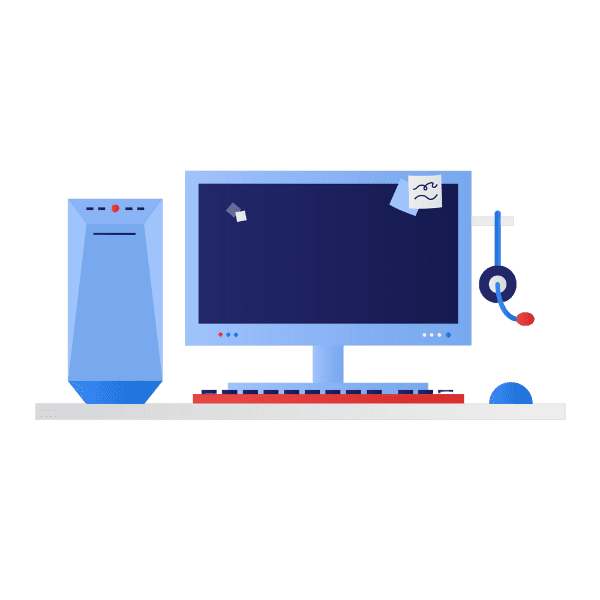
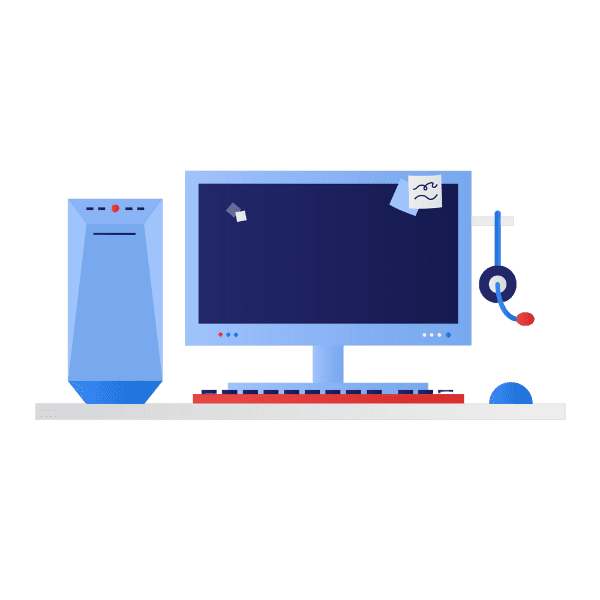
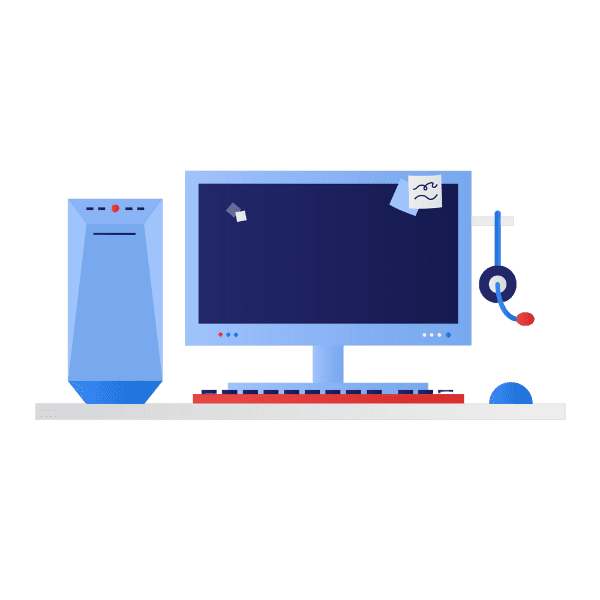
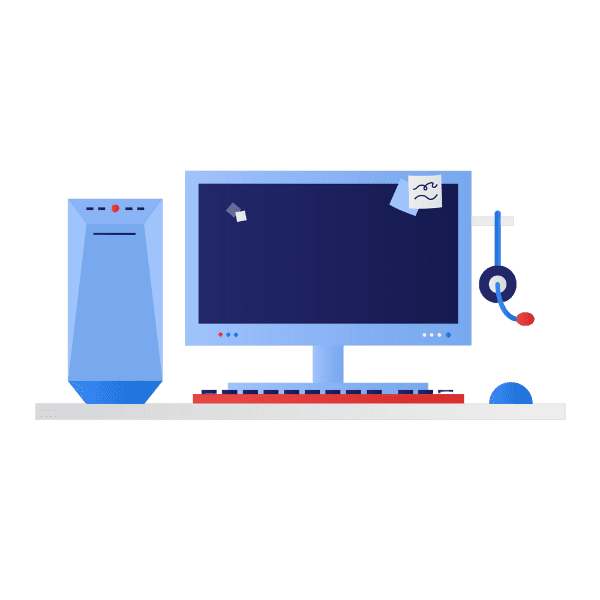
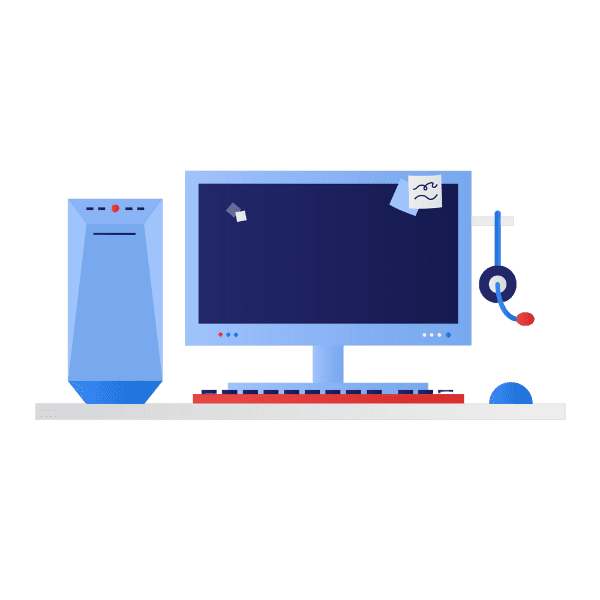
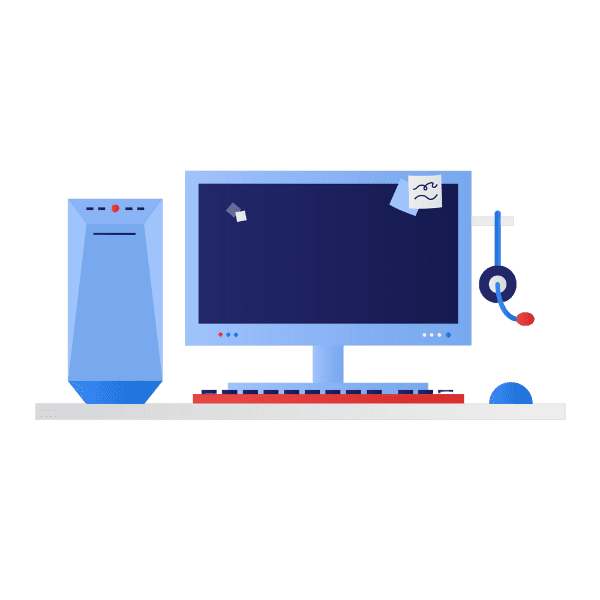
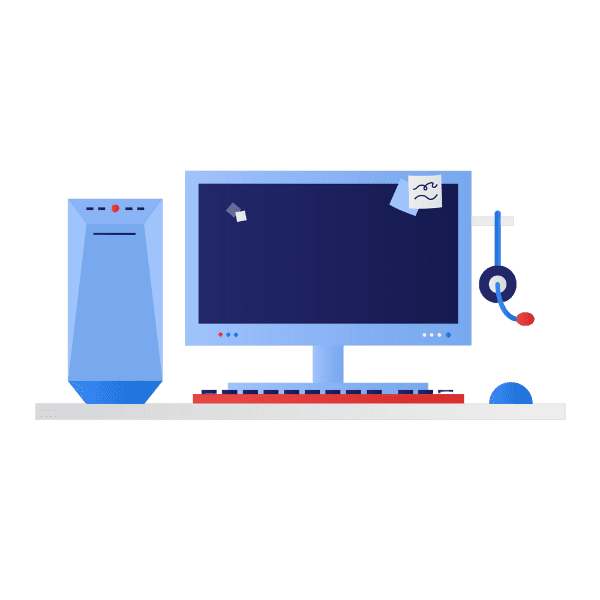
O Acer Nitro 5 AN515-58-58W3 brilha entre os notebooks com Linux, especialmente por sua impressionante taxa de atualização, tornando-o uma escolha ideal para gamers e profissionais que necessitam de precisão visual sem atrasos.
Esta máquina é equipada com uma tela de alta definição que suporta uma taxa de atualização elevada, permitindo que imagens e vídeos sejam exibidos de forma mais fluida e com menos arrasto, um fator crucial para jogos de alta velocidade e tarefas que exigem grande precisão gráfica.
Além disso, o Nitro 5 vem com componentes de hardware de ponta, incluindo um processador robusto e uma GPU dedicada, que trabalham em conjunto para suportar os requisitos de sistemas operacionais baseados em Linux, mesmo durante o uso de aplicativos intensivos em recursos.
Este notebook não só promete um desempenho gráfico excepcional, mas também oferece uma experiência de usuário suave e responsiva, graças à combinação de sua poderosa configuração interna e suporte ao Linux, garantindo que os usuários tenham à disposição uma máquina versátil e capaz de enfrentar uma ampla gama de desafios.
5 - Acer Aspire Nitro 5 AN515-47-R5SU
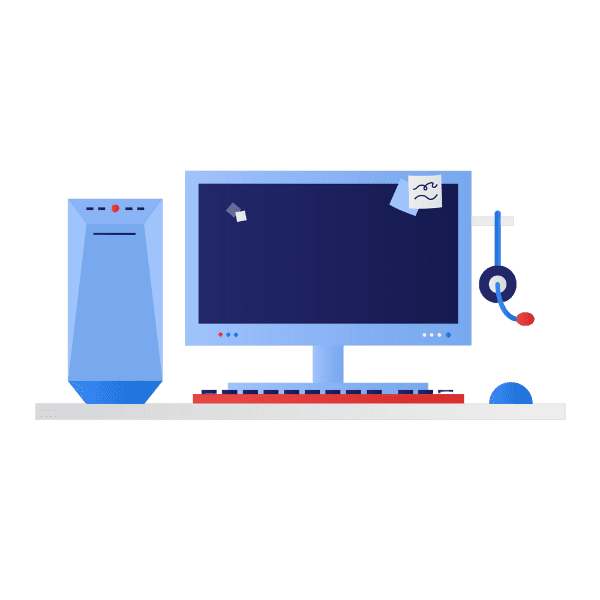
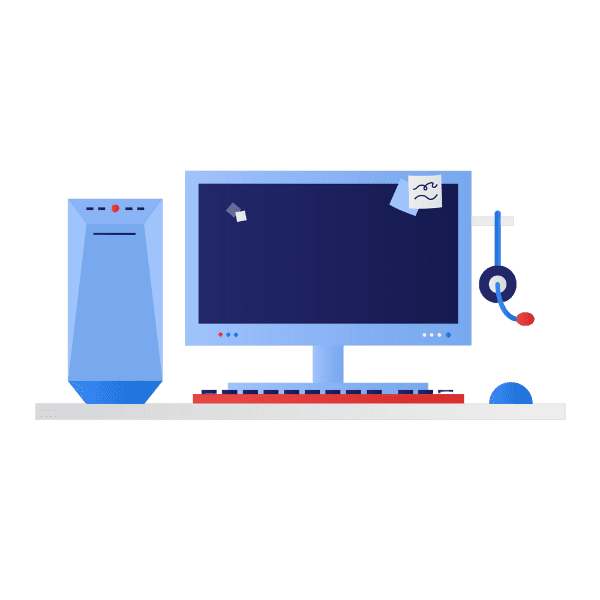
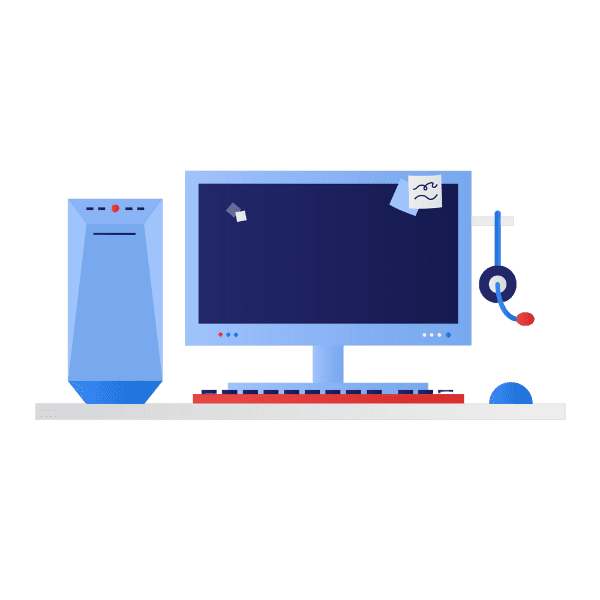
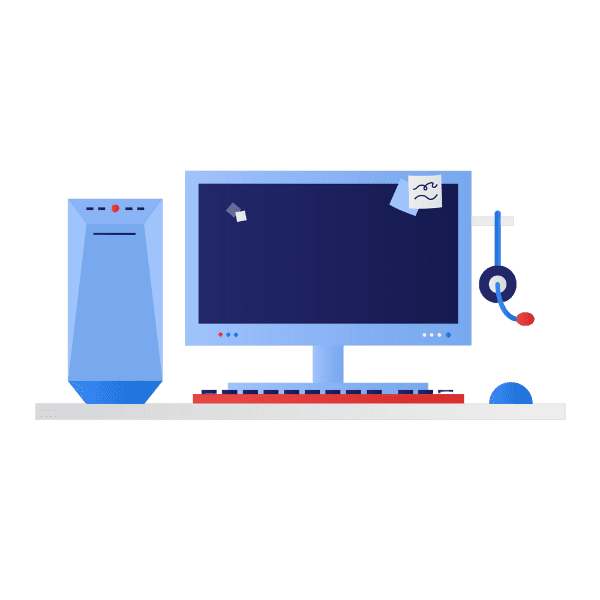
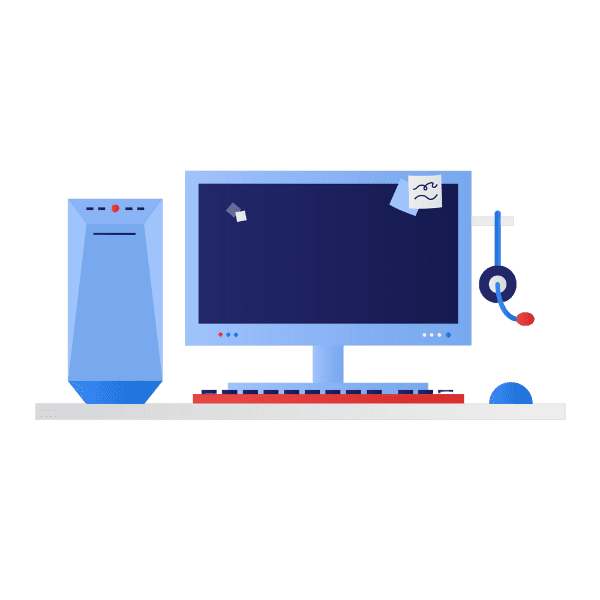
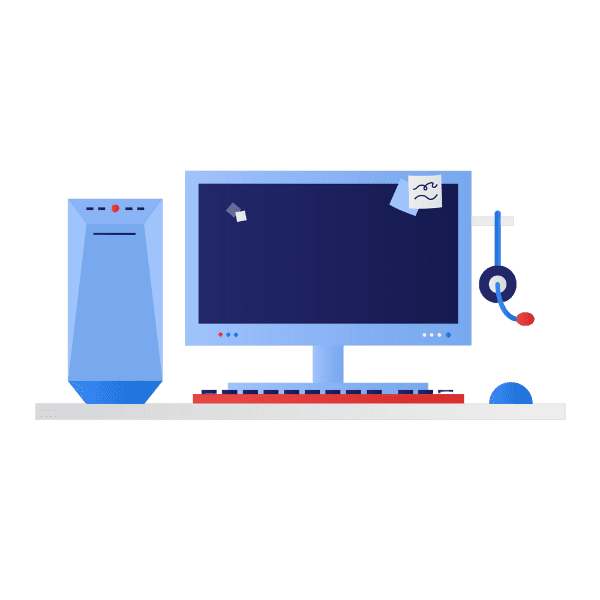
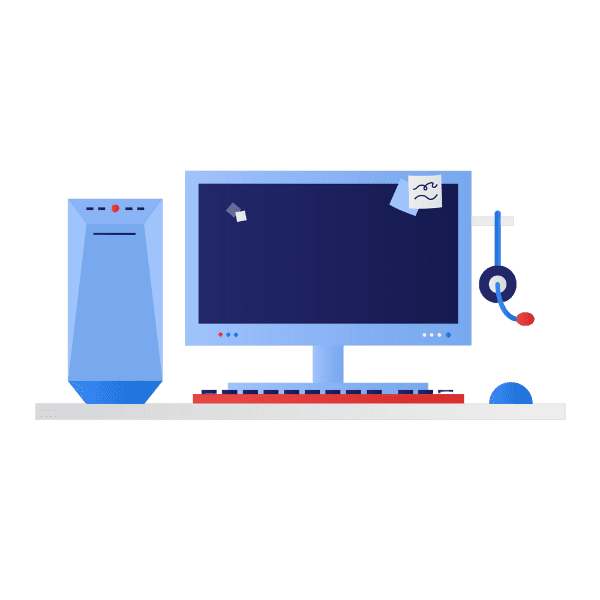
O Acer Aspire Nitro 5 AN515-47-R5SU se destaca por seu processador AMD que alcança até 4,5GHz, oferecendo desempenho excepcional para jogos, edição de vídeo e tarefas de computação intensivas. A alta velocidade do processador garante que o sistema opere de maneira fluida e responsiva, mesmo sob carga pesada.
Além disso, a compatibilidade com Linux deste modelo amplia as opções de personalização e segurança para os usuários. Esse notebook é ideal para quem busca alto desempenho e valoriza a flexibilidade do Linux.
6 ao 10 Posição
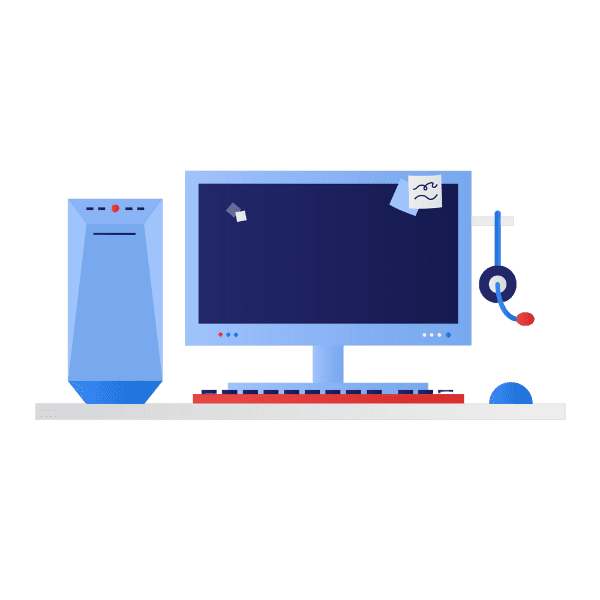
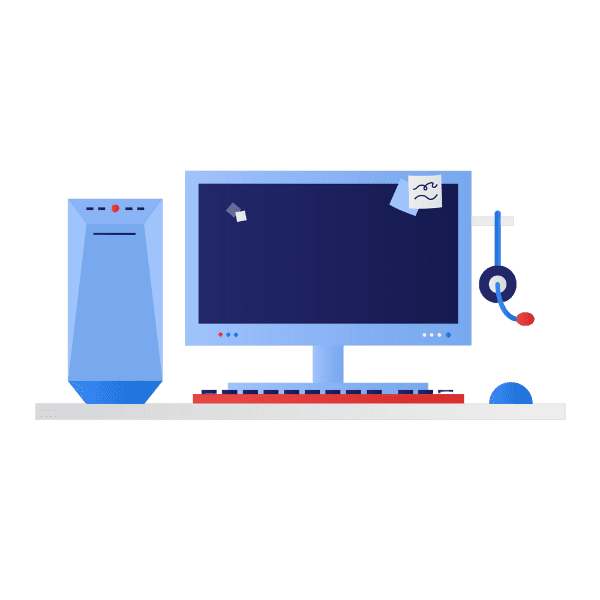
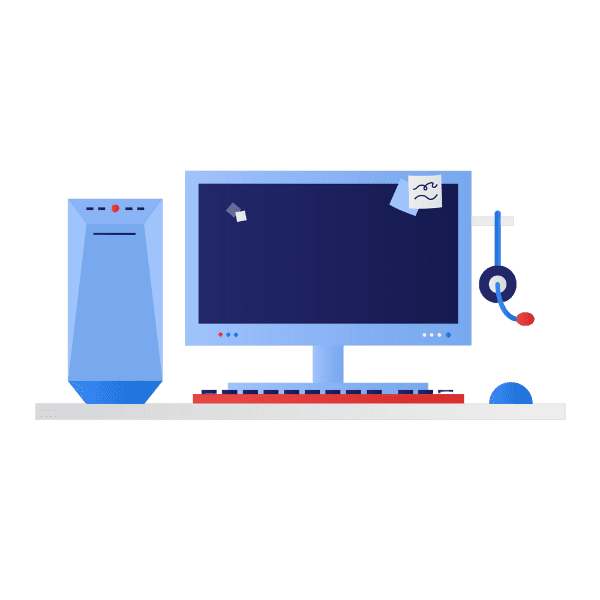
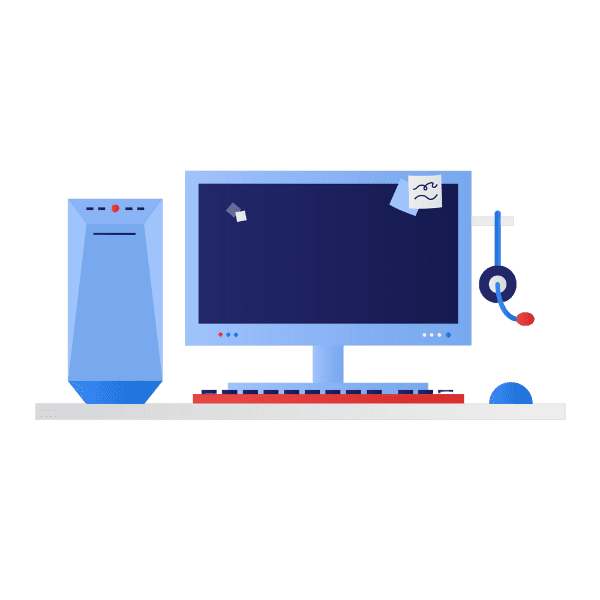
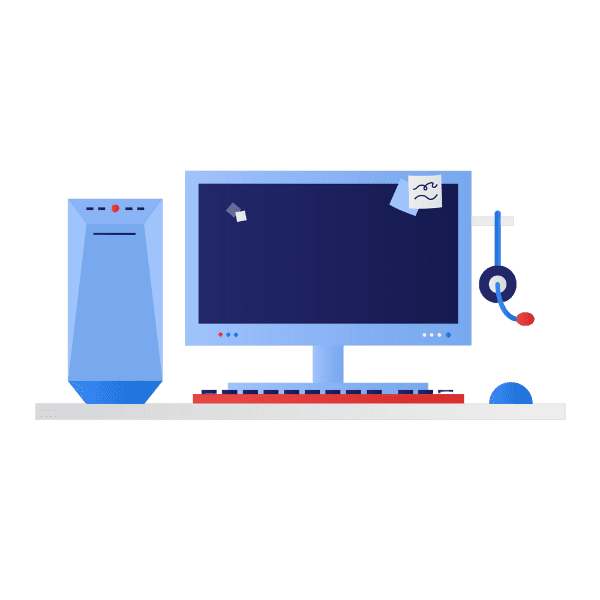
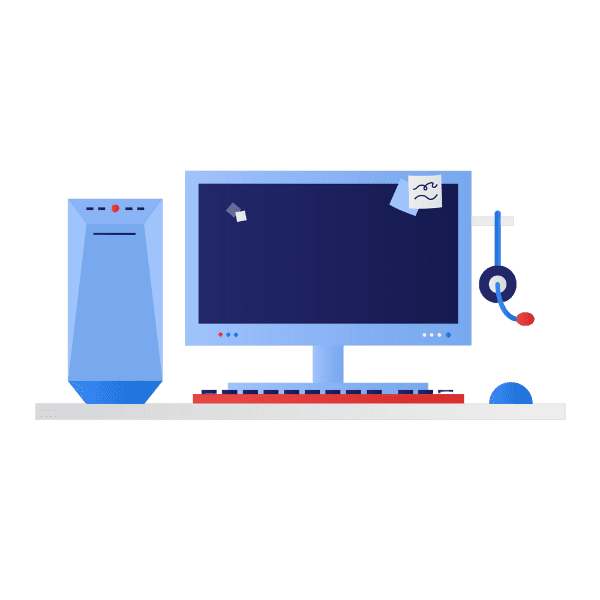
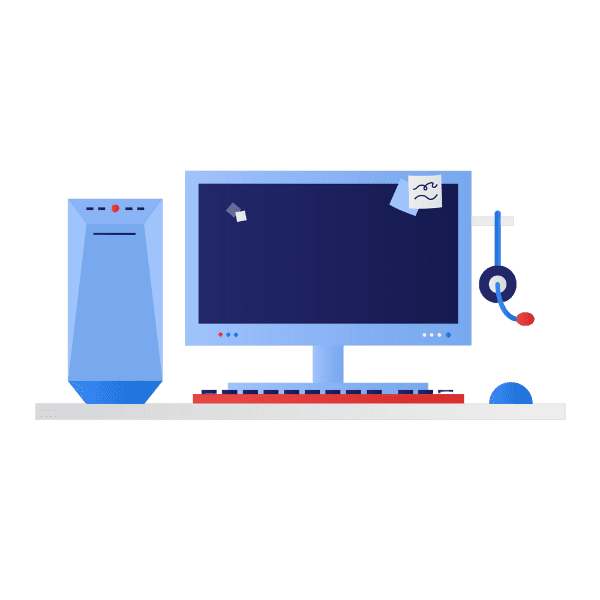
Dell G15 5530 G15-i1300-U20 – Intel de última geração.
Asus TUF Gaming F15 FX507ZC4-HN112 – design industrial.
Dell G15 5530 G15-i1300-U30 – notebook linux completo.
HP Pavilion Gaming 15-dk1056wm – Excelente para multitarefas.
Lenovo Legion 5 15ACH6H – Otimizado para jogos.
Melhor Notebook Linux em 2024: 10 Modelos com o Sistema Operacional
À medida que avançamos em 2024, o Linux continua a ser uma escolha popular de sistema operacional para muitos usuários, desde entusiastas de tecnologia e desenvolvedores até aqueles preocupados com privacidade e segurança. A flexibilidade, segurança e liberdade que o Linux oferece, combinadas com seu custo geralmente mais baixo em comparação com outros sistemas operacionais, fazem dele uma opção atraente para uma ampla gama de pessoas. Reconhecendo a crescente demanda por notebooks que ofereçam suporte nativo a este sistema operacional livre e aberto, várias marcas de renome lançaram modelos impressionantes que prometem tirar o máximo proveito do Linux. Neste artigo, destacamos 10 dos melhores notebooks Linux disponíveis em 2024, incluindo nossa escolha principal, o Asus VivoBook 16 X1605ZA-MB310, entre outros modelos que se destacam por seu desempenho, durabilidade e compatibilidade com o Linux. Se você é um desenvolvedor, um profissional de TI, ou simplesmente alguém que prefere a segurança e a customização que o Linux oferece, este guia irá ajudá-lo a encontrar o notebook perfeito para atender às suas necessidades em 2024.
Por que o Linux é Gratuito?
A gratuidade do Linux está profundamente enraizada em sua filosofia original e na decisão de Linus Torvalds, seu criador, de disponibilizar o código-fonte do Linux sob a GNU General Public License (GPL) no início dos anos 90. Esse ato não apenas definiu o curso do Linux como um projeto de software livre e aberto, mas também estabeleceu um modelo para a colaboração e inovação coletiva. A GPL garante que qualquer pessoa possa modificar e redistribuir o código-fonte do Linux, desde que as alterações permaneçam sob a mesma licença. Isso criou um ecossistema onde desenvolvedores de todo o mundo contribuem para o projeto, enriquecendo o Linux com suas habilidades e experiências diversas.
A natureza gratuita do Linux também é um reflexo do movimento de software livre, que valoriza a liberdade do usuário em executar, copiar, distribuir, estudar, modificar e melhorar o software. Esse movimento, liderado por figuras como Richard Stallman, não se concentra apenas na gratuidade do software em termos de custo, mas na liberdade que ele proporciona aos usuários. O Linux, como um pilar desse movimento, é gratuito para encorajar a inovação aberta, permitindo que empresas, instituições de ensino e indivíduos em todo o mundo utilizem, modifiquem e compartilhem software sem restrições.
Além disso, a gratuidade do Linux contribuiu para sua adoção generalizada em diversos setores da indústria e da tecnologia. Servidores, supercomputadores, dispositivos móveis, e sistemas embarcados frequentemente utilizam o Linux devido à sua estabilidade, segurança e flexibilidade. A capacidade de personalizar e otimizar o sistema para diferentes casos de uso sem incorrer em custos de licença torna o Linux particularmente atraente para startups e empresas que buscam soluções tecnológicas eficazes e econômicas.
Por fim, a gratuidade do Linux fortalece sua comunidade global de usuários e desenvolvedores. Uma vasta rede de suporte, fóruns, documentações e tutoriais estão disponíveis sem custo, tornando mais fácil para novos usuários aprenderem e se adaptarem ao sistema. Essa comunidade não apenas resolve problemas e desenvolve novas funcionalidades, mas também garante que o Linux permaneça acessível a todos, independentemente da capacidade financeira. A decisão de manter o Linux gratuito reflete um compromisso contínuo com os princípios de liberdade e colaboração, fundamentais para o crescimento e a inovação constantes do sistema operacional.
Como escolher o melhor PC Linux ?
Escolher o melhor PC Linux requer uma avaliação cuidadosa de suas necessidades específicas, pois o sistema operacional Linux é conhecido por sua versatilidade e capacidade de rodar em uma ampla gama de hardware. Primeiramente, é importante considerar o propósito principal do computador. Se você planeja usá-lo para tarefas intensivas, como desenvolvimento de software, edição de vídeo ou jogos, você precisará de um PC com especificações de hardware mais robustas, incluindo um processador potente, uma boa quantidade de memória RAM e, possivelmente, uma placa de vídeo dedicada. Por outro lado, para uso geral, como navegação na web, edição de documentos e mídia social, um modelo mais básico e econômico pode ser suficiente.
Ao escolher um PC Linux, também é crucial verificar a compatibilidade do hardware com o sistema operacional. Embora a maioria dos componentes de hardware modernos seja compatível com o Linux, alguns dispositivos específicos podem exigir drivers proprietários ou não oferecer suporte completo. Realizar uma pesquisa prévia para garantir que o hardware escolhido seja bem suportado pela distribuição Linux de sua preferência pode economizar tempo e evitar frustrações futuras. Felizmente, muitas comunidades e fóruns Linux oferecem informações detalhadas e suporte sobre compatibilidade de hardware.
Outro aspecto importante a ser considerado é a escolha da distribuição Linux, pois cada uma oferece diferentes ambientes de desktop, conjuntos de software pré-instalados e recursos de sistema. Distribuições como Ubuntu e Fedora são conhecidas por sua facilidade de uso e suporte amplo, tornando-as opções ideais para novos usuários ou aqueles que preferem uma experiência mais simplificada. Para usuários avançados ou aqueles que desejam uma customização profunda, distribuições como Arch Linux ou Gentoo podem ser mais atraentes. A escolha da distribuição pode influenciar a decisão sobre o hardware, pois algumas são mais leves e podem funcionar melhor em PCs mais antigos ou com recursos limitados.
Por fim, considere o suporte e a comunidade em torno do PC Linux que você está escolhendo. Uma comunidade ativa pode ser um recurso inestimável para resolver problemas, encontrar dicas de otimização e descobrir novos usos para o seu PC. Além disso, fabricantes que oferecem suporte explícito ao Linux ou vendem PCs com o Linux pré-instalado podem fornecer uma experiência mais integrada e sem problemas. Levando em conta esses fatores — finalidade do uso, compatibilidade de hardware, escolha da distribuição e suporte da comunidade —, você estará bem equipado para escolher o melhor PC Linux que atenda às suas necessidades e preferências.
Qual a Melhor Distribuição Linux para Cada Usuário em 2024
Melhores Distribuições Linux para Servidor Virtual Privado (VPS)
Na escolha da distribuição Linux ideal para um Servidor Virtual Privado (VPS), diversos fatores devem ser considerados, incluindo estabilidade, segurança, suporte e facilidade de uso. Algumas distribuições se destacam nesse cenário, cada uma oferecendo características únicas que podem atender a diferentes necessidades e preferências.
1. Servidor Ubuntu
O Ubuntu Server destaca-se no mundo dos servidores VPS pela sua excepcional facilidade de uso, robusta segurança e estabilidade inigualável.
Com um ciclo de lançamento regular que garante atualizações de segurança contínuas e um vasto repositório de pacotes, o Ubuntu Server é adaptável tanto para usuários iniciantes quanto para profissionais experientes de TI. Seja para hospedagem de websites, execução de serviços baseados em nuvem ou desenvolvimento de aplicações web complexas, o Ubuntu Server oferece uma base sólida com o apoio de uma extensa comunidade online. Essa comunidade é uma fonte rica de documentação e suporte, facilitando a resolução de problemas e a implementação de novas funcionalidades.
2. Red Hat Enterprise Linux (RHEL)
O Red Hat Enterprise Linux representa a escolha premium para ambientes VPS, graças à sua incomparável segurança de nível empresarial, robustez e suporte completo. Ideal para ambientes de missão crítica, o RHEL proporciona uma plataforma confiável para o desenvolvimento e execução de aplicações empresariais. O modelo de assinatura do RHEL inclui não apenas o suporte abrangente da Red Hat, mas também acesso a uma vasta gama de softwares e atualizações. Adicionalmente, usuários do RHEL beneficiam-se do acesso a inovações através do Fedora Project e do CentOS Stream, garantindo que estejam sempre na vanguarda das tecnologias open source. Empresas que necessitam de uma solução VPS confiável com amplo suporte e recursos avançados de segurança encontrarão no RHEL uma opção sem igual.
3. Rocky Linux
Após a mudança de direção do CentOS, o Rocky Linux rapidamente se estabeleceu como uma alternativa viável e confiável, projetada para oferecer estabilidade e compatibilidade binária com o RHEL, sem os custos associados às licenças empresariais. Voltado para a comunidade, o Rocky Linux apela para aqueles que procuram uma distribuição confiável para seus servidores VPS, ideal para hospedagem de websites, servidores de aplicativos e ambientes de desenvolvimento. Sua compatibilidade com o RHEL assegura que os usuários podem desfrutar de uma plataforma estável e segura, com a vantagem de ser totalmente suportada por uma comunidade ativa e engajada. Para desenvolvedores e empresas que buscam uma solução econômica sem comprometer a qualidade e a estabilidade, o Rocky Linux emerge como uma escolha sólida e promissora.
Qual a diferença de um PC Linux para outros?
A distinção entre um PC Linux e outros sistemas operacionais reside fundamentalmente na filosofia, estrutura e liberdade que o Linux oferece. O Linux, sendo um sistema operacional de código aberto, permite que usuários e desenvolvedores tenham acesso completo ao seu código-fonte. Essa característica fundamental promove uma cultura de transparência, inovação contínua e colaboração comunitária, algo que sistemas operacionais proprietários como Windows e macOS limitam a um certo grau. A liberdade de modificar e distribuir o código do Linux não só fomenta um ambiente de personalização sem precedentes, mas também facilita a criação de uma variedade de distribuições (distros) Linux, cada uma com seu próprio conjunto de características, interfaces de usuário e foco de aplicação, desde desktops e servidores até sistemas embarcados.
Em termos de segurança, os PCs Linux são amplamente reconhecidos por sua robustez. O modelo de segurança do Linux é projetado com múltiplas camadas de proteção, o que, combinado com atualizações frequentes da comunidade e revisões rigorosas do código, torna o sistema operacional menos suscetível a vírus e malwares. Esta vantagem de segurança é acentuada pela menor quota de mercado do Linux em desktops, tornando-o um alvo menos lucrativo para os criadores de malwares em comparação com sistemas operacionais mais populares. Além disso, a natureza aberta do Linux significa que vulnerabilidades, quando descobertas, são geralmente corrigidas rapidamente pela comunidade ou por contribuidores corporativos.
Do ponto de vista da performance, PCs Linux podem ser significativamente mais rápidos e eficientes do que seus equivalentes em outros sistemas operacionais, especialmente em hardware mais antigo ou de especificações mais baixas. Isso se deve em parte à leveza de muitas distribuições Linux, que podem funcionar com requisitos mínimos de sistema, revitalizando PCs antigos que lutariam para rodar versões mais recentes de sistemas operacionais proprietários. Além disso, a capacidade de personalizar o sistema operacional permite que usuários avançados e administradores de sistemas otimizem seus PCs Linux para desempenho máximo, seja para computação de alto desempenho, desenvolvimento de software ou jogos.
Finalmente, a adoção de um PC Linux pode significar uma redução de custos significativa. Além do sistema operacional ser gratuito, a maioria do software disponível para Linux também é de código aberto e gratuito. Isso inclui uma ampla gama de aplicativos produtivos, ferramentas de desenvolvimento, programas gráficos e jogos. Para empresas e instituições educacionais, isso pode representar economias substanciais em licenças de software. Além disso, a comunidade Linux oferece suporte extensivo através de fóruns, guias online e documentação detalhada, proporcionando uma rede de suporte valiosa para usuários de todos os níveis de habilidade. Esses aspectos, combinados, tornam os PCs Linux uma opção atraente para uma variedade de usuários, desde entusiastas de tecnologia e profissionais de TI até organizações focadas em custo e segurança.